- StudySolver
- /Population Of United States
Population Of United States
Introduction
The population of United States is aging. Between now and 2030 the number of adults aged 65 and older is projected to reach 72.8 million or 20 percent of the U.S population, more than double the population of 35 million older adults in 2000 (Hetzel & Smith, 2000; Ortman, Velkoff, & Hogan, 2014). As these numbers increase, so do concerns about society’s ability to meet the social and economic needs of older adults, particularly the growing number of those with few, if any, financial resources beyond Social Security in retirement. Concerns are warranted. The two major components of the U.S retirement income system, Social Security and employer-based defined pension plans, have undergone significant changes in recent years prompting researchers and legislators to encourage older adults to delay retirement, work longer and take greater personal responsibility for their own economic well-being in retirement. These efforts are based largely on projections that demographic shifts resulting in a disproportionate number of older adults in the labor market will expand opportunities for older adults to work longer as businesses experience critical labor shortages (Abraham & Houseman, 2008; McEvoy, & Blahna, 2001). However, in spite of projections, research suggests that many older adults looking for work, particularly those who have experienced an involuntary job loss, face significant challenges to reemployment that may induce them to withdraw from the labor market even when it is not economically feasible to do so (Shultz, Morton, & Weckerle, 1998; van Solinge & Henkens, 2007). Although research exists exploring the labor force behavior of older adults after an involuntary job loss, this research typically emphasizes transitions to other states of employment (i.e., employment, unemployment, labor force withdrawal) and subsequent financial outcomes. Far less, however, is known about whether disparities exist in the pathways taken by subgroups of older adults. Without systematic efforts to understand how diversity influences the labor force behavior of older unemployed adults, interventions cannot be tailored to the needs of those most vulnerable in the labor market and efforts to encourage working longer will result in ongoing issues with early labor force withdrawal and income insecurity in late adulthood.
The purpose of this research is to examine the labor force behavior of older adults after an involuntary job loss and to test the effects how individual characteristics, including gender, race, and education, influence transitions to full and part-time employment. To this end, I begin with a discussion of the importance of working longer for older adults before reviewing the relevant literature on labor force transitions among older adults. Afterwards, I discuss the significance of this issue for social work practice and research and conclude with a description of my proposed study. Background
Historically, older adults have relied on income from Social Security and employer-based defined benefit pension plans (DB) to maintain their standard of living in retirement (Quinn & Cahill, 2015; Rhee, 2013; Segal, 2014). Social Security is an employment-based social insurance program that promises older workers replacement income when they are no longer able to work (Sass, 2003). Contributions are proportionate to a worker’s earnings up to a maximum amount and benefits paid are based on earnings and work history (Sass, 2003). Because most workers in the U.S. are covered by Social Security, it is unsurprising that 84 percent of older adults received benefits in 2014 (Social Security Administration, 2016). However, what is surprising is the 61 percent of beneficiaries relying on Social Security for more than half their income and the 33 percent relying on benefits for 90 percent or more of their income in retirement (Segal, 2014; SSA, 2016). While Social Security is an important source of income, older adults were never intended to rely primarily on benefits in retirement (Segal, 2014). Indeed, to maintain standards of living in retirement, the average older adult needs to replace roughly 85 percent of their pre-retirement income (Rhee, 2013). Under the current benefit formula, Social Security only provides a replacement rate of roughly 35 percent for the average household, leaving a retirement gap of 50 percent of pre-retirement earnings (Rhee, 2013). Further, political opposition threatens the future solvency of the Social Security Program (Quinn & Cahill, 2016; Segal, 2014; Toossi, 2015). If no action is taken, Social Security’s combined Old-Age and Survivors Insurance (OASI) and DI trust funds will be exhausted in 2034 (Board of Trustees, 2016). After 2034, Social Security would only be able to pay three-fourths of scheduled benefits (Board of Trustees, 2016).
Besides Social Security, employer-based retirement plans have historically provided the most important source of income for older adults in retirement. However, a declining share of workers have access to employer based retirement plans; among those with access to plans through an employer, DB plans are much less likely to be offered (Rhee, 2013; Rhee, 2014; Rhee & Boivie, 2015). More specifically, nearly 55 percent of private sector workers have access to retirement plans at work with only 4 out of 10 being covered by DB plans (Rhee & Boivie, 2015). DB plans provide older adults with a guaranteed level of income in retirement based on their years of service, age at retirement, and level of income over the working years (Rhee, 2013). While DB plans are provided by private companies, the federal government insures them through the Pension Benefit Guaranty Corporation (PBGC). The maximum pension benefit guaranteed by PBGC is set by law and adjusted yearly. For plans that end in 2016, the maximum guarantee for workers who retire at age 65 is $60,136 yearly ($5,011.36 monthly) (PGBC, 2016). The guarantee is lower for those who retire early or when there is a benefit for a survivor; the guarantee is increased for those who retire after age 65 (PGBC, 2016). Despite the financial security offered, shifts among companies in the private sector have resulted in fewer workers having access to DB plans (Rhee, 2013). Instead, those with access to employer sponsored retirement plans must take on the financial risks and responsibilities associated with defined contribution (DC) plans which require employees to contribute a portion of their income to an individual retirement account (Rhee, 2013). Employers may also make contributions or match the employee’s contribution, but DC plans are uninsured and do not provide a guaranteed level of retirement income (Rhee, 2013). Instead, they rely on private investment programs such as stock market based mutual funds to create income growth (Rhee, 2013). As DB plans have become much less prevalent than DC plans, much of the funding burden and investment risk of retirement fall on individuals, who tend to have to difficulty contributing enough on their own and who typically lack investment expertise (Rhee, 2013). As a result, the shift from DB pensions to DC plans has significantly eroded the retirement readiness of many older adults.
In response to concerns of income security in retirement, policymakers have taken several important steps to encourage older adults to work longer and reverse trends toward early retirement or retirement that occurs before the age of full eligibility for retirement benefits, which is between the ages of 65 or 66 depending on one’s date of birth (Abraham & Houseman, 2008). For example, recent legislative changes allow older adults receiving full retirement benefits to work without their benefits being reduced and permit workers under the normal retirement age to phase into retirement while working a reduced schedule (Abraham & Houseman, 2008). Further, benefits have been made less generous by increasing the age at which workers may collect full Social Security benefits (National Academy of Social Insurance, 2016). Subsequently, increases in labor force participation among older adults have been noted over the last several decades (Bureau of Labor Statistics (BLS), 2008). Still, many who would benefit from working longer continue to retire early (Quinn & Cahill, 2016). This is particularly disconcerting, as research suggests that a significant number of older adults who would benefit from working longer, but choose to retire, do not have the financial resources to maintain their standard of living through retirement (Munnell, 2014a; Munnell, 2015; Quinn & Cahill, 2016, Rhee, 2013). Indeed, Poterba, Venti, and Wise (2012) found that nearly half of older adults outlive their financial assets and must rely primarily on Social Security in late life. Further, approximately 64 percent of older adults draw their Social Security retirement benefits before reaching the full retirement age (FRA); resulting in permanent reductions to their monthly benefits; up to 25 percent of those born between 1943 and 1954, and up to 30 percent of those born after 1959 (Munnell, 2015; Quinn & Cahill, 2016; U.S. Social Security Administration, 2015). Working until FRA would add significantly to their lifetime monthly benefit payments in retirement. Additionally, for each year older adults can delay retirement beyond the FRA, their Social Security payment increases by 8 percent (Munnell, 2015; Quinn & Cahill, 2016). The difference between claiming retired worker benefits at age 66 and age 70 is an incredible 32 percent increase in monthly benefits (Munnell, 2015).
Statement of the Problem
While working longer offers significant financial benefit to older adults, it is based upon the assumption that opportunities to work are available to those interested in doing so. However, the economic reality, is rarely, if ever, this simple (Krugman, 2009). The demand for labor changes frequently and the ability to work can be interrupted for reasons unrelated to job performance. For example, when businesses incorporate the use of technologies that eliminate or reduce the need for workers or changes in the demand for goods and services result in mass layoffs, involuntary job loss and subsequent unemployment are often the result. Historically, involuntary job loss has been concentrated in the goods-producing sector of the economy as companies restructured to compete with global competitors (Figura & Wascher, 2008; Kalleberg, 2008). In recent years, workers in the service sector, who were once largely shielded from the effects of involuntary job loss, are now increasingly susceptible to its effects as our economy continues to change (Kalleberg, 2008). Although many workers find employment after an involuntary job loss, older workers face greater difficulties in our changing labor market. In addition to the immediate financial consequences associated with being unemployed including the loss of income and an inability to continue preparations for retirement, older workers lose their job tenure and the job-related skills they have acquired may become obsolete (Brand, 2015). As a result, older workers frequently encounter longer durations of unemployment and lower job quality and substantial wage loss upon reemployment (Farber, 1993; Farber, 1997; Farber, 2011). Further, those near retirement age may exit the labor force and withdraw retirement benefits to offset financial strain imposed by prolonged unemployment even when it is not in their best interest to do so (Chan & Stevens, 2002). Although substantial research exists documenting the effects of involuntary job loss on subsequent wage loss and employment outcomes, unprecedented growth in diversity among older adults necessitates ongoing research to determine how race, gender, and education influence labor force behavior among older adults after involuntary job loss.
Significance of the Study
This research is significant in that it attempts to answer calls for research on unemployment in the social work literature and reassert the professions commitment to research surrounding issues related to unemployment. A lot of what has been published and is accessible on unemployment in the social work literature was written in the 1980s through the early 1990s and argues for greater social work involvement with the unemployed. For example, Briar (1980, 1983) calls for greater involvement of social work in unemployment issues and discusses unemployment as a source of client problems as well as suggesting strategies for helping the unemployed client, their families, and communities; Sherraden (1985a, 1985b) brings conceptual clarity to issues surrounding unemployment by distinguishing the various forms of unemployment and suggesting social work responses to chronic unemployment; McKay, Berglind, Dhooge, Popay & Pilcher (1990) examine social works unresponsiveness to the needs of the unemployed and proposes a number actions the profession can take to increase and strengthen social work’s knowledge and skills for working with and on behalf of the unemployed; Beckett & Root (1990) focus on unemployment caused by plant closing and discusses the individual, family, community, and societal consequences of plant shutdowns in the United States, Canada, and Europe and discuss ways that social work can expand their current practice domains to meet the needs of displaced workers, their families, and communities. Calls for greater social work involvement in unemployment issues have more recently come from Reisch and Gorin (2001) and Goldberg (2012) who detail changes in the U.S economy since the 1970s, governmental policies that support economic inequality, and an increasing need for social workers to be concerned with a wider range of policy and advocacy issues. Further, as researchers and policymakers encourage working longer as a way to assuage issues related to income security in retirement, it is important that social workers understand and document the challenges of those most vulnerable in the labor market and advocate for programs and services that support their continued employment.
Theoretical Framework
A life course perspective is helpful in examining the powerful connection between individuals and the historical and socioeconomic contexts in which their lives unfold. As a concept, a life course is defined as "a sequence of socially defined events and roles that the individual enacts over time" (Giele and Elder 1998, p. 22). These events and roles do not necessarily proceed in an ordered sequence, but constitute the sum of an individual’s experiences. Concepts central to an understanding of the life course perspective include: cohort, life events, transitions, and trajectories (Elder, 1985). A cohort refers to a group of persons born during the same historical time who experience social changes in the same sequence and at the same age (Hutchison, 2005). For example, baby boomers, those born between 1946 and 1964, represent a particularly large cohort of individuals whose development has been marked by dramatic social change (Schuman & Scott, 1989). Their experiences have made them culturally distinct from their parents and their children. Life events refer to significant occurrences involving abrupt change that may produce serious and long lasting effects (Hutchinson, 2005). Life events often result in transitions or changes in status, social identity, and role involvement within a trajectory or long-term pattern of stability and change (Hutchinson, 2005). For example, involuntary job losses are abrupt life events that often result in changes to an individual’s employment state; they transition from being employed to being unemployed. The changes involved in transitions are discrete and bounded; when they happen, one phase ends and a new phase begins. In contrast, trajectories involve a longer view of long-term patterns of stability and change in a person’s life, involving multiple transitions (Elder & Kirkpatrick Johnson, 2003; George, 2003; Heinz, 2003).
Several dominant, and interrelated, themes have been identified in the life course approach. They include: the interplay of human lives and historical time, the timing of lives, linked or interdependent lives, diversity in life course trajectories and developmental risk and protection. The interplay of human lives and historical contexts refers to the ways in which lives are interdependent and connected across systems. Macro and micro-level experiences are linked through the family and its network of shared relationships (Elder 1998). As a result, macro-level events affect individual behavior and this can significantly affect other relationships within families. For example, stressful events, such as job loss, also affect family relationships because these occurrences trigger patterns of stress and vulnerability or, conversely, promote adaptive behaviors and family resilience (Elder, 1998). The interdependence of human lives refers to the ways in which relationships are linked and support or control individual behavior through expectations, rewards, and punishment (Hutchinson, 2005). Further, families frequently coordinate their lives with regard to life planning and matters related to the timing of life events. This sometimes generates tension and conflict, particularly when individual goals conflict with needs of the family as a collective unit (Hutchinson, 2005). The timing of lives refers to the age or developmental stage at which specific events and transitions occur (Hutchinson, 2005). Entrances and exits from a particular status or role can be classified as off-time or on-time based on social norms or shared expectations about the timing of social transitions (George, 1993). For example, early retirement or retirement occurring before the age of 62 is frequently regarded as off-time because social norms suggest retirement transitions typically occur between the ages of 62 and 65. Diversity in life course trajectories, another important tenet of the life course approach suggests that one must consider variability in trajectories that exists within cohorts (Hutchinson, 2005). Research supports the notion that individuals within cohorts have different experiences, and helps to overcome the notion that cohorts share similar perspectives because they share a common age group (Riley, 1987). Indeed, cohorts are not homogeneous collections of people. Rather, they differ in terms of influential dimensions such as gender, social class, family structure, ethnicity, and religion. Moreover, the ability to adapt to changes across the life course can vary with the resources or supports inherent in these elements in the form of economic capital (i.e., wealth, education) or social capital (i.e., family social support). Literature review
Between January of 1980 and July 1980, the U.S. entered a recessionary period caused by an increasing federal funds rate. As credit became more difficult to obtain, severe contractions in manufacturing and housing resulted in significant job loss in the manufacturing and construction industry, while employment in the service sector remained relatively unaffected. Although this recessionary period officially ended in July 1980, the downturn did not end until sixteen months later in November 1982. Over one million jobs were loss from the manufacturing sector with the recession exhibiting a total loss of 1.3 million jobs; remaining job loss occurred predominately in the construction industry (Gardner, 1994). The automotive industry, already in a poor position at the time due to weak sales in 1979, shed 310,000 jobs; 33 percent of employment in that industry. Similarly, the construction industry shed approximately 300,000 jobs (Gardner, 1994). Before the economy could fully recover, the U.S entered another recessionary period between July of 1981 and November 1982 as ongoing weakness in manufacturing and construction began to effect economic growth in the service sector of the economy (Bednarzik, Hewson, & Uruquhart, 1982). Overall, this recessionary period resulted in the loss of an additional 2.9 million jobs from the economy (Gardner, 1d994).
The U.S enjoyed robust job growth and declining employment rates prior to the early 1990s recession, however, between July 1990 and March 2001 the economy shed approximately 1.6 million jobs mostly in the construction and manufacturing industries (Gardner, 1994). Job loss during this recessionary period disproportionately affected specific regions of the country. Among the hardest hit regions were the New England states and the West Coast, while the Midwest and South Central states were less affected (Dzialo, Shank, & Smith, 1993). Further, in March 2001, the U.S entered a global recession that lasted 8 months and resulted in the loss of 1.7 million jobs in 2001 and an additional loss of 508, 000 jobs in 2002 (Klieson, 2003).
The most recent recessionary period, often referred to as the Great Recession occurred from December 2007 to June 2009. Deeper and more extensive than any other recessionary period since the Great Depression, the U.S. unemployment rate fluctuated between 9 to 10 percent between 2009 and 2011; the highest rate since the early 1980s (Hout, Levanon, & Cumberworth 2011). Of the of 8.8 million jobs loss, 2.0 million were from the manufacturing industries (Goodman & Mance, 2011). Further, the manufacturing industry is expected to lose an additional 814, 000 jobs by 2024 (Henderson, 2015).
Economic Effects of Job Loss
When workers experience involuntary job loss, there are immediate financial challenges associated with the loss of income; particularly for workers unable to quickly secure new positions with comparable wages and benefits. Although unemployment benefits help assuage financial strain experienced by displaced workers, benefits typically only replace up to 46.3 percent of lost wages and with dramatic disparities existing from state-to-state (Department of Labor Employment & Training Administration, 2015). For example, the benefit can replace as little as 34.6 percent of a lost earnings in Alaska to as much as 56.3 percent of a lost earnings in Hawaii (DOLETA, 2015). Further, benefits typically last for only 21 weeks (DOLETA, 2015). This poses significant financial problems for workers experiencing involuntary job loss considering the average displaced worker experiences long durations of unemployment (Brand 2004; Chan & Stevens 1999; Farber 2003, 2005; Kletzer 1998). Even when workers do not experience long durations of unemployment, they often suffer substantial earning losses (Kletzer 1998; Brand 2004; Stevens 2014; von Wachter 2010). Couch and Placzek (2010) suggest that following an involuntary job loss, there is an immediate 33 percent earnings loss and a loss of as much as 15 percent six years following job separation. The cumulative lifetime earning loss is estimated to be roughly 20 percent, with wage scarring (residual effects of earning loss) observed as long as 20 years after displacement (Brand and von Wachter 2013; Davis and von Wachter 2012; von Wachter 2010; von Wachter, Song, and Manchester 2009). Further, reemployed displaced workers are more likely than their non-displaced counterparts to be employed part-time, and this likelihood has increased in recent years, particularly during recessions (Farber 1993b, 2003, 2005). Displaced workers may also find, when they are reemployed, that their jobs are of lower quality in terms of job authority, autonomy, and employer-offered benefits in comparison to both the jobs they lost and those held by their non-displaced counterparts (Brand 2004, 2006; Podgursky & Swaim 1987). Workers may also endure greater job instability for at least a decade following a displacement event (von Wachter, 2010).
While economic losses occur for displaced workers across demographic categories, across industries and throughout the skill distribution (von Wachter, 2010), effects vary by worker characteristics. Displaced workers’ losses reflect both industry-specific decline and the loss of firm-specific and industry-specific skills (Kalleberg, 2000). Workers experiencing involuntary job loss from jobs where they held higher tenure, those who change industries, and those who experience multiple job losses thus experience greater earnings losses (Carrington & Zaman, 1994; Couch, Jolly, & Placzek 2009; Fallick, 1996; Stevens, 1997; von Wachter, 2010; Farber, 2011). This is particularly problematic because, while displacement is painful for all workers, it poses additional challenges for older workers. Older workers who experience involuntary job loss are less likely to be reemployed, have less time to adjust their retirement plans, and are more likely to retire prematurely (Mandall & Roe, 2008; Sum, Trubsky, Khatiwada, McLaughlin, & Palma, 2015). With a contracting retirement income system and increased life expectancy, the continued employment of older workers is increasingly critical to older workers’ ability to ensure a secure retirement.
Factors Associated with Involuntary Job Loss
As noted above, substantial research exists documenting the effects of worker displacement on subsequent wage loss and employment outcomes. However, because the nature of involuntary job losses often changes based upon economic context in which they occur, it is difficult to develop policy and interventions that address the unique needs of those most vulnerable to displacement in any given period. Indeed, as worker displacement and associated periods of unemployment have become more common, subgroups of the population have been disproportionately affected. For example, although women and Whites were significantly impacted by displacement in the 1980s, males, particularly African American and Hispanic males had higher probabilities of being displaced. During the 1990s, the incidence of involuntary job loss increased among women and Whites, as well as for college-educated workers and those with higher tenure on their pre-displacement jobs (Couch 1998; Farber 1993b, 1997, 2005). Additionally, disadvantaged family background, employment in manufacturing jobs, low occupational status, low job tenure (less than 3 years on the job), and low levels of education heightened the risk of job loss over this period (Brand 2005; Farber 2005). While workers with higher levels of education typically maintain a lower risk of displacement, increased rates of displacement for this group, from 5.4 percent between1987 to 1989 to 11.0 percent between 2007 to 2009, have aroused public concern that the structure of job loss has qualitatively changed over recent decades, increasing vulnerability to job loss across the population (Farber, 2011).
Gaps in the Existing Literature
Although ample research exists examining labor force transitions among older adults following involuntary job losses, much of the extant literature focuses on the short-term and long-term earning losses associated with reemployment following an involuntary job loss. Distinctions are rarely made about whether these outcomes reflect transitions to full- or part-time employment. Thus, more research differentiating the likelihood of transitions to full-time employment versus part-time employment is needed to document potential problems with underemployment among displaced older workers, particularly among those of pre-retirement age. The extant literature is also outdated and does not reflect current cohorts of older displaced workers, more specifically, baby boomers. The life course perspective suggests inter-cohort differences may exist in the likelihood of transitions to employment following an involuntary job loss largely influenced by the different sociohistorical contexts of school and work trajectories for baby boomers and older cohorts displaced of workers (i.e., baby boomers are likely to have more education resulting in more career options than earlier cohorts of older adults); previous research may not reflect the experiences of older displaced baby boomers. Also, relatively few studies examining labor force transitions among older displaced workers explore the extent to which diverse characteristics, such as race, ethnicity, gender, and education influence labor force transitions following involuntary job loss and subsequent unemployment. This is needed to help further our understanding of those least likely to find new jobs following involuntary job losses.
Further, although labor shortages resulting from significant growth in the older adult population relative to the working age population are projected to create an unprecedented demand for older workers, these projections seem to contradict research that suggests many older adults face significant challenges finding new jobs after involuntary job loss. This may largely be a function of low demand for skills associated with occupations with higher incidences of displacement. More research is needed to explore the effects of prior employment experiences on likelihood of reemployment.
Purpose of the Study
The purpose of this study is to examine labor force participation among older adults; to explore the incidence of involuntary job loss among older adults; and to test the influence of gender, race, and education on the likelihood of reemployment among displaced older adults.
Research Questions and Hypotheses
The proposed study addresses the following research questions:
1. How does gender influence labor force transitions among older unemployed adults?
2. How does race influence labor force transitions among older unemployed adults?
3. How does education influence labor force transitions among older unemployed adults?
Methods
I propose to conduct a secondary analysis of the Health and Retirement Study longitudinal dataset. The major advantages associated with secondary analysis are the cost effectiveness and convenience it provides (Dale et al., 1988; Glaser, 1962; Smith, 2008). Since the data has already been collected, financial resources are not needed for data collection. Secondary data analysis also allows access to high quality datasets, such as those collected by the HRS involving larger samples and contain substantial breadth. The larger sample sizes afforded by the HRS are more representative of the target population and allow for greater validity and more generalizable findings (Smith, 2008; Smith et al., 2011). However, there also unique methodological considerations when utilizing existing data to investigate new research questions and generate new knowledge. The most recognized limitation to secondary data analysis is “inherent in its nature” in that the data were collected for some other purpose (Boslaugh, 2007, p. 4). Since the data was not collected to answer the specific research questions, issues may arise. For example, it would be ideal for this research if data were collected at more frequent intervals. This would add valuable information on the duration of unemployment among older adults and more nuanced transitions that might not be captured during data collection. For example, an older adult who is unemployed in wave 1, but becomes employed and unemployed again before the next wave of data collection has transitioned in and out of employment; this information is valuable, but multiple labor force transitions would be difficult to capture with the current data. With that being said, the HRS questionnaire does provide detailed information on most variables of interest in this research including respondent’s work history as well as demographic, economic, and job characteristics. It is, therefore, adequate for use in this study.
Information about the Data
The HRS is a longitudinal dataset of older Americans that began in 1992. The initial set of “Core” HRS respondents, those aged 51 to 61 in 1992, and their spouses consisted of 12,652 respondents from approximately 7,600 households. Interviews have been conducted every other year since 1992 with additional cohorts added in 1998 (the “War Babies,” born from 1942 to 1947), in 2004 (the “Early Baby Boomers,” born from 1948 to 1953), and in 2010 (the “Mid Baby Boomers,” born from 1954 to 1959). Data was originally collected from HRS respondents in one of two ways: through a face-to-face interview, or through an interview with a proxy. Later waves of data collection were completed using telephone follow-up (Smith et al., 2001). With the goal of making the data more accessible, the RAND Center for the Study of Aging, with funding and support from the National Institute on Aging (NIA) and the Social Security Administration (SSA), created the five data products including the RAND HRS Data File (v.O), a user-friendly file derived from all waves of the HRS; RAND Enhanced Fat Files which contain most of the original HRS variables with household data merged to the respondent level; RAND Income and Wealth Imputation File (v.O) which contains the component and ownership variables that were used in RAND HRS income and wealth summary measures; RAND Family Data Files derived from HRS other person files; and the RAND CAMS Spending Data File (v.D2) a user-friendly version of the spending section (Part B) of the CAMS survey.
Sample
Sample selection will proceed in two steps. First, the RAND dataset variable RwUNEMP will be used as an indicator of whether respondents are considered unemployed as defined by the Bureau of Labor Statistics (BLS). This definition considers those who are not working, but actively seeking work as unemployed. The derivation uses questions about current work status, work for pay, and activities related to job seeking. If the respondent is working for pay, then RwUNEMP is set to employed (0=No). If the respondent is not working for pay and reports actively looking of a job in the last four weeks, RwUNEMP is set for unemployed (1=yes). In 2010, 899 respondents were unemployed. Next, the 2010 RAND Enhanced Fat File dataset variable MJ073M1, which asks about the reasons for unemployment, will be used to further delimit the sample to respondents who indicate being unemployed due to business closures or layoffs. In the 2010 wave, 146 respondents indicated they were unemployed due to business closures. An additional 475 indicated being unemployed because of layoffs.
Independent Variables
Race. Race refers to a respondent’s self-reported racial identification. It will be measured with the RAND HRS dataset variable RARACEM where 1= white, 2=black, and 3=other.
Ethnicity. Ethnicity refers to whether respondents identify themselves as Hispanic. It will be measured with the RAND HRS dataset variable RAHISPAN where 0=not Hispanic and 1=Hispanic.
Gender. Gender refers to a respondents self-reported biological sex. It will be measured with the dataset variable RAGENDER where 1= male and 2=female.
Age. Age refers to the chronological age of respondent in the 2010 wave. It will be measured with the RAND HRS dataset variable R10AGEY_E which is specified in years based on the birthdate of a respondent and the ending interview date.
Education. Educational refers to highest level of education attained by respondents and measured by the RAND HRS dataset variable RAEDUC. If the Respondent has a high school diploma or GED and over 12 years of education, RAEDUC is set to "some college." RAEDUC is also set to "some college" if the degree is less than a BA, or "other." If the Respondent has exactly 12 years of education but no college degree, then a high school diploma is assumed. If the college degree is a BA or greater, RAEDUC is set to "college and above." Respondent has a high school diploma or GED and years of education over 12, RAEDUC is set to "some college." RAEDUC is also set to "some college" if the degree is less than a BA, or "other." If the Respondent has exactly 12 years of education but no college degree, then a high school diploma is assumed. If the college degree is a BA or greater, RAEDUC is set to "college and above."
Marital status. Marital status refers to whether respondents considered themselves to be married at the time of interview. It will be measured with the HRS RAND dataset variable MA026 which asks respondents whether they are married with their responses being coded as 1=yes and 2=no.
Health. Health refers to the Respondent’s self-reported general health status. It is measured with the dataset variable R10SHLT and codes range from "1" for Excellent to "5" for Poor.
Industry. Industry refers to sector of the economy respondents report they are employed. It will be measured with the HRS RAND dataset variable R10JCIND and is specified as: 01= Agriculture/Forestry/Fishery; 02= Mining and Construction; 03= Manufacturing: Non -durable goods; 04= Manufacturing: Durable goods; 05=Transportation; 06=Wholesale; 07= Retail; 08=Finance/Insurance/Real Estate; 09= Business/Repair Service; 10= Personal Service; 11= Entertainment/Recreation; 12= Professional/Related Services; 13= Public Administration.
Dependent Variables
Transition to employment. The primary dependent (outcome) variable is labor-force transition between the two waves. For this study, a labor force transition is defined as a change in respondent’s labor force status from unemployed to either full or part time employment. For this study, labor force transition will be operationalized as self-reported changes in labor force status from unemployed in 2010 to employed full or part-time in 2012. To determine an individual’s labor force status in 2012, responses to the HRS RAND dataset variable RwLBRF will be used as an indicator of whether respondents are now employed. RwLBRF summarizes the labor force status for the Respondent at each wave where 1=full-time, 2=working part-time, 3=unemployed, 4=partly retired, 5=retired, 7=disabled, or 7=not in the labor force. The RAND HRS derivation uses many different questions that are available each wave. A Respondent can give evidence of working, being retired, and disability alone or in combination with other statuses. RwLBRF attempts to pull information from several sources in the original HRS data, and sort through the discrepancies. Working and retirement take precedence in its derivation. However, because the interest in this study is whether respondents transitioned into employment in 2012, responses will be recoded with working full-time and working part-time being indicative of transitions into employment and all other response categories being indicative of no transition into employment.
Statistical Analysis
The primary analysis will rely on multinomial logit (MNL) model to examine the influence of race, gender, and education on the likelihood of transitions to employment among older displaced workers. Multinomial Logistic Regression is useful for situations in which you want to be able to classify subjects based on values of a set of predictor variables. This type of regression is similar to logistic regression, but it is more general because the dependent variable is not restricted to two categories. It performs a series of binary logistic regressions, comparing each categorical value against each other and the reference category. In this analysis, the dependent variable, labor-force transition, is a discrete variable that takes three unordered and independent outcomes: (1) working full-time, (2) working part-time, and (3) no transition into employment.
Implications for Social Work
As the population ages, social workers will increasingly be called upon to address the economic needs of older adults in an environment where benefits are less generous and older adults are expected to assume much of the responsibility for their own retirements. As working longer will increasingly be prescribed to remedy financial need, it is important to expand our understanding of barriers to remaining in, reentering, or staying out of the workforce for older adults. Although personal income, access to pensions, and macroeconomic factors likely play central roles for those displaced from jobs, we are likely to find that their labor force decisions are nuanced and multifaceted. For example, some older adults may become discouraged and drop out of the labor force, while others leave because flexible schedules are unavailable. Whatever their reasoning, social workers are needed to advocate for older adults and the extension of programs and services that assist older adults interested in work.
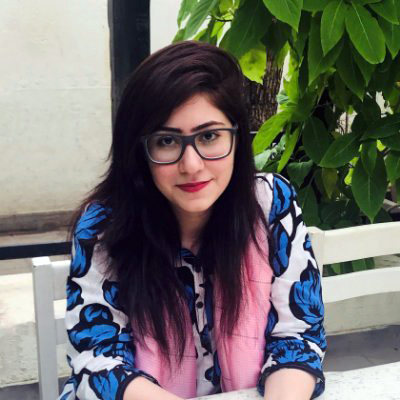
Freelance Writer
I’m a freelance writer with a bachelor’s degree in Journalism from Boston University. My work has been featured in publications like the L.A. Times, U.S. News and World Report, Farther Finance, Teen Vogue, Grammarly, The Startup, Mashable, Insider, Forbes, Writer (formerly Qordoba), MarketWatch, CNBC, and USA Today, among others.