- StudySolver
- /Other Expansions Of The Dyadic Data
Other Expansions Of The Dyadic Data
4.4. Other expansions of the Dyadic Data
By incorporating features of both the APIM and the CFM in the same model, which is referred to as hybrid dyadic model [19], researchers can investigate associations between both personal-level and common fate variables. For example, these models are capable of testing hypotheses regarding whether a common fate variable influences partners’ individual variable. The hybrid models also can be expanded to examine mediating and moderating mechanisms in dyadic data [19, 21, 22].
Kenny and Garcia [34] extended the APIM to the study of groups, and they called this extended model the group APIM (GAPIM). This model can be used to assess the extent to which predictors of interest exhibit homogeneity and to estimate the effect of homogeneity on one’s attitudes, perceptions, and/or behaviors. General reviews and applications of the GAPIM have been given by Garcia et al. [35] Ervin and Bonito [36].
All of the models presented so far in this article are designed for quantitative outcome variables. However, many studies in the field of behavioral medicine have categorical outcome variables. To cover the categorical outcome variables in the APIM, researchers present some approaches to estimate actor and partner effects based on generalized estimating equations (GEE) [37]. Details of the data analysis, and SAS syntax have been given in Refs [37, 38].
5. Conclusion
Dyadic models provide researchers an interesting way to study interpersonal processes. The inclusion of both members of dyad in the data set provides the opportunity to not only correct bias in the estimates, but to study dyadic nonindependence. The aim of this article was to introduce the researchers to dyadic models and its applications especially in behavioral medicine research. We hope that our review encourages researchers to learn more about dyadic data designs, particularly APIM, and how to apply them within the context of behavioral medicine. We still feel that existing dyadic models need to be extended further to address effectively both intrapersonal and interpersonal process regarding health and well-being. Furthermore, the integration of the dyadic models, particularly APIM, with other analytic statistical methods has the potential to address principal and complicated questions about health behavior.
Over the past decade, there has been an increasing interest in dyadic data analysis across health sciences. For health scientists, the theory of dyadic data has been a gold mine, yielding explanations for intrapersonal and interpersonal processes of health in various types of dyads, including husband-wife, caregiver-patient, parent-child, siblings and friends. Most studies in this context, with a dyadic nature, use the individual as the unit of analysis. Although valuable, these studies fail to take into account the non-independency of data, and consequently would lead to biased results. Nowadays, these dyadic designs are being used increasingly in behavioral medicine as a framework for investigating interpersonal processes. Nevertheless, many of researchers still require more information on how to analyze the study’s data in a way that optimizes its value. Therefore, the aim of this article is threefold. First, we explain how dyadic conceptualization can aid our understanding of dyadic nature of phenomena in behavioral medicine. Second, we review three methods and their extensions for analyzing dyadic data: Actor-Partner Interdependence Model, Common Fate Model, and Mutual Influence Model. Third, we provide a comprehensive review of the conceptual examples that have utilized these models to demonstrate how these dyadic techniques could be utilized to answer questions in health study.
1. Introduction
Over the past decade, there has been a great deal of interest in dyadic data analysis. This type of data arises naturally in many fields, including medical, behavioral, psychological, and social sciences [1-3]. In these fields, many of the phenomena studied by researchers are dyadic in nature and interpersonal by definition. People involved in dyadic relationships may affect each other’s thoughts, emotions, and behaviors, which leads to interdependence in a relationship [1, 4]. Interdependence refers to circumstances in which the characteristics of one member of the dyad affects outcomes of the other member in the dyad [1, 4]. This occurs in a variety of contexts that involve two individuals, such as relationships between husband-wife, doctor-patient, caregiver-patient, parent-child, siblings and friends [1, 5]. For example, a husband’s/wife’s depression can affect his/her partner’s quality of life. In other words, the husband’s/wife’s depression does not only affect his/her own quality of life (intrapersonal effects), but also his/her partner’s quality of life (interpersonal effects).
Dyadic data analysis treats the dyad, rather than the person, as the unit of analysis; however, in this case independence refers to independence from dyad to dyad. Conventional statistical techniques are not proper for analyzing dyadic data; Instead, the techniques that take into account the interdependence is required [1, 5].
Most studies in this behavioral medicine, with a dyadic nature, use the individual as the unit of analysis. Although valuable, these studies fail to account for interdependence of data, and consequently would lead to biased results [5]. However, in recent years an increasing number of scientists have started to develop dyadic data analysis methods that properly take into account interdependence, which cannot be completely acknowledged with individualistic designs [2, 3]. Nowadays, these dyadic designs are being used increasingly in behavioral medicine as a framework for investigating interpersonal processes. Nevertheless, many of researchers still require more information on how to analyze the study’s data in a way that optimizes its value [3]. Finally, use of dyadic data analysis techniques gave the researchers a better understanding of interdependent mechanisms that contribute to health studies. Therefore, the aim of this article is threefold. First, we explain how dyadic conceptualization can aid our understanding of dyadic nature of phenomena in health study. Second, we review three methods for analyzing dyadic data: Actor-Partner Interdependence Model, Common Fate Model, and Mutual Influence Model. Third, we provide a conceptual and statistical example to demonstrate how these dyadic techniques could be utilized to answer questions in health study.
2. Basic Terms in Dyadic Data Analysis
There are a variety of key terms that should be considered when employing dyadic data designs in behavioral medicine.
2.1. Distinguishability
On important issue in dyadic research is whether or not dyad members are distinguishable [1, 5]. This issue plays a crucial role in determining the proper data analytic approach and must be assessed both conceptually and empirically. Conceptually, dyad members are said to be distinguishable if the two can be differentiated on a nonarbitrary variable and indistinguishable if they cannot. Example of distinguishable dyads are heterosexual couples or patient-caregiver in which members of dyads are distinguished by variables gender and role, respectively. Alternatively, homosexual couples, identical twins, and same sex roommate are all example of indistinguishable dyad. Empirical distinguishability refers to whether the conceptual distinguishability actually matters in terms of observed differences in means, variances, and covariances for dyad members’ scores as a function of the distinguishing variable. Comprehensive information about testing distinguishability is available elsewhere [6, 7].
2.1. Nonindependence
Two members of a dyad share something in common that we refer to as nonindependence. This issue is the most fundamental concept for dyadic data analysis, and sometimes indicates that persons are interdependent [1]. Conceptually, interdependence refers to circumstances in which the characteristics of one member of the dyad affects outcomes of the other member in the dyad [1, 4]. This issue generates the need for specific statistical treatments of the observations. Investigations have shown that ignoring the fact that data are interdependent may lead to biased results.
2.3. Type of Dyadic Variables
Like the concept of distinguishability, the nature of the independent variable also plays a crucial role in determining the proper data-analytic approach to study dyads. There are three main classes of variables in dyadic data analysis: between-dyad variables, within-dyad variables, and mixed variables [1]. The nature of the independent variable plays an important role in determining the appropriate data-analytic approach for dyadic data. Scores on a between-dyads variable differ from dyad to dyad, but not within a dyad, and so both members have same scores on the variable (e.g. length of a couple’s marriage). Within-dyad variables are variables that vary exclusively within dyad but not between dyad when aggregated. With this type, the dyad average is constant across dyads (e.g. gender in heterosexual couples). Dyad members are differentiated by a within-dyads variable. Mixed variables are variables that vary both within dyads and between dyads (e.g. quality of life and depression). Most commonly used outcome variables in dyadic studies are mixed.
2.4. Types of Dyadic Designs
There are three main type of dyadic designs: standard dyadic design, one-with-many design, and social relation model (SRM) design [8]. As presented in Figure 1, the key difference between these models is the way the dyad members are paired together. In the standard dyadic design, each individual is paired with one, and only one, other individual (e.g. married couples). In the one-with-many design, one individual (i.e., the focal individual) is paired with multiple others, but these others (i.e., the partners) are not paired with any other individuals (e.g. doctor with his patients). In the SRM design, every person is paired with multiple other persons (e.g. team or family members rating one another). The most frequently used design, which we also focus on in this review, is the standard dyadic design [3].
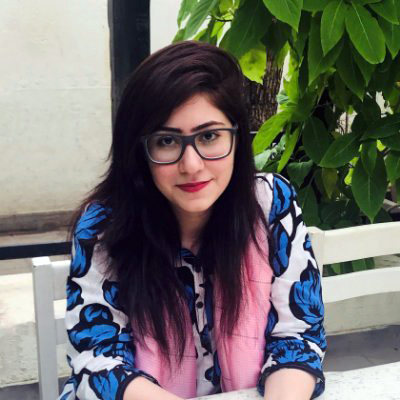
Freelance Writer
I’m a freelance writer with a bachelor’s degree in Journalism from Boston University. My work has been featured in publications like the L.A. Times, U.S. News and World Report, Farther Finance, Teen Vogue, Grammarly, The Startup, Mashable, Insider, Forbes, Writer (formerly Qordoba), MarketWatch, CNBC, and USA Today, among others.