- StudySolver
- /Internal Management Practices (Independent Variable)
Internal Management Practices (Independent Variable)
Internal management practices (Independent Variable)
For the statistical analyses of the second hypothesis, the research study will incorporate two of the four management practice categories of the World Management Survey and a subgroup of the additional items both identified by Bloom and Van Reenen (2007). More specifically, the items include the categories associated with Targets (6 items), Incentives (5 items) and the supplementary Central (3 items) information, while the analyses exclude the items related to Operations and Monitoring. Due to restructuring of the survey questions relative to the original survey set by Bloom and Van Reenen (2007), the research study derives the principal components of the incorporated 14 items to retrieve the management practices categories with a specific focus on the human capital aspect. The results indicate four factors with an Eigenvalue greater than 1, which cumulatively explain 61.4% of the total variance. Following an oblique rotation of the loadings, I label these four factors Management Score in Human capital, Management Score in Decision Making, Management Score in Target Coverage and Management Score in Target Setting. Further, I provide additional details about individual item loadings in the Appendix I.B – Table 3. The focus, however, will be on the “Management Score in Human capital” variable and its relation to voluntary disclosure. Nonetheless, the remaining latent variables serve as potential control variables to account for the majority of the internal practices.
Guidance (Dependent Variable)
To account for potential guidance provision to external stakeholders by the organizations, this dummy variable equals 1 if a management forecast with an earnings-per-share (EPS) measure is issued during the fiscal year t, and 0 otherwise.
Forecast Error (Dependent Variable)
This variable accounts for the absolute difference between the forecasted EPS by management and the actual EPS. Following Karamanou and Vafeas (2005), with the aim to ensure consistent comparisons across organizations, this measure is scaled by the logged assets per share at the beginning of the fiscal period.
Forecast Bias (Dependent Variable)
Like the forecast error variable, this measure accounts for the difference between the forecasted EPS by management and the actual EPS, however, not in absolute but signed terms. Further, a positive (negative) value suggest forecast optimism (pessimism). Lastly, this measure is also scaled by the logged assets per share at the beginning of the fiscal period (Karamanou and Vafeas, 2005).
Miss (Dependent Variable)
This measure accounts for meeting/missing the forecast targets in terms of point estimates (all retrieved EPS targets are illustrated as point estimates). The respective dummy variable equals 1 if the organization achieves an actual EPS greater than the forecasted EPS in the managerial guidance, and 0 otherwise.
Systematic Risk (Control Variable)
This variable accounts for the potential market-wide risk effects, as firms with higher risk levels are more inclined to provide additional guidance to the external market. The construction of the variable is based on the standard deviation of predicted returns at the beginning of the year, following the market returns over an estimation period of 12-60 months (Kasznik, 1995).
Analyst Disagreement (Control Variable)
This control aims to incorporate the interanalyst uncertainty in the earnings forecast of a firm (Brown, Foster and Noreen, 1985). In other words, management most likely experiences difficulties with respect to earnings forecasting when the outcome of this variable is higher and, subsequently, may face greater litigation risk (Brown, Foster and Noreen, 1985). The measure is defined as the standard deviation of analysts’ forecasts divided by the median forecast.
Earnings Volatility (Control Variable)
Like Analyst Disagreement, Earnings Volatility aims to account for fluctuations in the earnings and future prospects. Reason for inclusion results from the established association between an organizations earnings volatility and the frequency of management earnings forecasts, which may bias the results (Waymire, 1985). The variable is computed based on the standard deviation of quarterly earnings over 12 quarters ending in the current fiscal year, divided by median asset value for the period (Ajinkya, Bhojraj and Sengupta, 2005).
Loss (Control Variable)
Further, the study also controls for organizational loss-making due to the specific implications this may cause to the external environment. For instance, previous research indicates that earnings have lower value-relevance for loss-making firms (Hayn, 1995). Furthermore, reaching financial analyst targets becomes less important and various authors established a significant difference between the analyst forecast errors of loss and profit firms. (Degeorge, Patel, and Zeckhauser, 1999; Brown, 2001). This indicator variable equals 1 if the organization reports losses in the respective fiscal year, and 0 otherwise.
Number of Analysts (Control Variable)
This measure captures the number of analysts following the organization. Past literature indicates a positive relationship between the quality of organizational disclosure and the number of analysts following the organization (Brown, Foster and Noreen, 1985). The resulting control is computed with the natural logarithm of one plus the number of analysts that issued an EPS forecast at the beginning of the fiscal year. However, this variable mainly influences the actual issuance compared to the forecasting properties. As a result, the subsequent regressions with respect to forecast properties include an alternative control variable (Horizon) (Karamanou and Vafeas, 2005).
Horizon (Control Variable)
This variable follows previous literature and attempts to account for greater earnings uncertainty and the unobservable accuracy of managers’ beliefs (Baginski and Hassell, 1997). The research study uses the common definition of the number of days between the forecast date and the end of fiscal period. As previously mentioned, the horizon variable is included due to the greater impact on the forecasting properties relative to Number of Analysts (Karamanou and Vafeas, 2005).
ROA (Control Variable)
To control for extreme operating performance, the study includes the ratio between the organization’s operating income divided by the total assets.
Methodology – Testing: Event Study (Hypothesis 1a)
To test these hypotheses, both proprietary data on organizational design choices and publicly available data of the award-winning firms and their respective corporate valuations are used. The initial sample consists of 168 corporations from numerous industries that received the ATD BEST Award between 2009-2014 (ATD BEST Awards, 2018). The event study analysis on this data is based on the framework outlined by Brown and Warner (1985) and the respective four steps for analyzing and evaluating the effects of events are performed.
1. Data Collection: The market returns, and adjusted closing prices are obtained from CRSP, Orbis and Compustat.
2. Specification of event date: The ATD BEST training award receipt day will be labeled as the event date with the event windows specification of ± three-, ± five-, ± ten- and ± fifteen-days.
3. Calculation of Expected Return (CAPM):
Ex(Rit) = αi + βi * RMarket + uit
Where Ex(Rit) is the expected return of organization i on date t, α is the intercept, βi is
the beta of organization i, RMarket is the market return (proxied by the S&P 500) on date t, and is the error term for firm i on event date t.
4. Calculation of Abnormal Return:
ARit = Ac(R it) – Ex(R it)
Where ARit is the abnormal return of organization i on date t, Ac(Rit) is the actual return of organization i on date t,
To further strengthen the statistical power, I conduct both parametric and non-parametric tests. More specifically, the study applies a Patell test (also standardized residual test), a standardized cross-sectional, a Rank test and a generalized Sign test with respect to the abnormal return estimation. The parametric tests allow to control for event-induced volatility and serial correlation (Patell, 1976; Boehmer, Musumeci and Poulsen, 1991). While the non-parametric tests enable the analysis of data, which may be subject to outliers or measured imprecisely, the main advantage lies in the avoidance of assumption making with respect to the parameters (Corrado and Zivney, 1992; Cowan, 1992). Following the robustness testing of return significance, the study attempts to explain the variation in these abnormal returns. With this intention, I conduct the subsequent regression analyses.
Methodology – Testing: Regression Analyses (Hypothesis 2a-2e)
The overall model of the subsequent regression follows below:
ARit = β0 + β1R&D Intensityit + β2Salary Competitivenessit + β3Marketing_Intit + β4Marketing Intensityit + β5PPE Intensityit + β6Firm Performanceit + β7Firm Sizeit + β8Previous Awardit + β9Industry Dummies + β10Country Dummies + εit (1)
The respective results are shown in Tables 15 and 17. Further, to identify the individual effects of the independent variables, I will test the respective variables in different models. Each of the respective models 2-7 (Model 1 used as the control model) is analyzed three times (Event day, ± Three-Day Event Window and ± Five-Day Event Window), and each version applies a different abnormal return window. The choice for the specific windows follows the findings in Hypothesis 1, the significance of the abnormal returns in these event windows and the support of these windows in previous research studies (MacKinlay, 1997). Further, the descriptive statistics are reported in Appendix II.A – Table 12.
Methodology – Testing: Probit Analyses (Hypothesis 3a-3c)
The model for Hypothesis H3a is shown below:
Pr(Issuance)it = β0 + β1Management Score in Human captialit + β2Management Score in Decision Makingit + β3Management Score in Target Coverage it + β4Management Score in Target Settingit + β5Firm Sizeit + β6Systematic Riskit + β7Analyst Disagreementit + β8Earnings Volatilityit + β9Lossit + β10ROAit + β11Previous Awardit + β12Number of Analystsit + εit (2)
The results of the guidance probability are shown in Table 20. Further, to identify the properties of the individual forecasts, I will test the different dependent variables in different models. The resulting three models are displayed below, and the results of their analyses are depicted in Table 21. As previously mentioned, in these models the Number of Analysts is replaced with the Horizon variable. Further, the descriptive statistics are reported in Appendix III.A – Tables 18 and 19.
The model for Hypothesis H3b-3c is shown below:
Forecast Biasit = β0 + β1Management Score in Human captialit + β2Management Score in Decision Makingit + β3Management Score in Target Coverageit + β4Management Score in Target Settingit + β5Firm Sizeit + β6Systematic Riskit + β7Analyst Disagreementit + β8Earnings Volatilityit + β9Lossit + β10ROAit + β11Previous Awardit + β12Horizonit + εit
(3)
Pr(Miss)it = β0 + β1Management Score in Human captialit + β2Management Score in Decision Makingit + β3Management Score in Target Coverage it + β4Management Score in Target Settingit + β5Firm Sizeit + β6Systematic Riskit + β7Analyst Disagreementit + β8Earnings Volatilityit + β9Lossit + β10ROAit + β11Previous Awardit + β12Horizonit + εit (4)
V. RESULTS AND DISCUSSION
This section displays the results of the statistical tests defined in the methodology part and describes the findings. Firstly, the study focuses on the findings of the event study and subsequent significance test. These significant results are then used to answer the first research question, which focuses on the economic value of human capital development. Next, the regression results with respect to the abnormal return variance are analyzed and described.
Results of Event Study
Hypothesis 1a
In general, the results of the parametric and non-parametric assessments indicate significant evidence to suggest that organizations derive economic returns from their expenditures into human capital development, as proposed by the first hypothesis (H1). The tests focus on numerous estimation windows including (-0, +0) to (-15, +15), which provide significant evidence for hypothesis 1 at the 10%, 5% and 1% significance levels. The respective reasons for these specific windows include the potential leakage of the award information for windows preceding the event date, while estimation periods following the event date aim to account for delayed trading and information-processing periods of stakeholders. This premise is supported by the results (Appendix II.B – Table 13 and 14) which indicate significant abnormal returns for the estimation windows (3) and (4) relative to the event window (5) estimation. In other words, the actual announcement day fails to fully impound the price changes. Furthermore, the longer estimation periods (1) and (2) provide non-significant abnormal returns, which could result from a dilution of information over the extensive period.
The findings result from the two commonly applied parametric tests, the Patell test and the standardized cross-sectional test, which solely differ in the correction method for potential cross-sectional variation (Patell, 1976; Boehmer, Musumeci & Poulsen, 1991). As a result, the discussion of the parametric test will focus on the estimations by the Patell test, however, the outcomes of the Standardized Cross-Sectional test provide similar results at slightly lower significance levels (Table 13). The first event period (-15, +15) shows a mean cumulative abnormal return (CAR) of 0.89%, which indicates that the average organization experienced an unexplained return of 0.89% over the 31-day event period. The respective Z-score based on a Patell estimation equals 0.791 and has a p-value of 0.2144. Consequently, this estimation window provides a positive but non-significant mean CAR. Similarly, the slightly shorter estimation period of 21-days (-10, +10) indicates a 0.77 mean CAR, which is represented by a Patell Z-score of 0.617. This estimate leads to a p-value of 0.2686 and, subsequently, also provides a positive but non-significant return. The estimation period regarding the 11-day event window suggests a 1.03% mean CAR (Patell-Z = 1.704, p-value= 0.0441), which follows the previous argument of higher returns around the announcement date. Further, this return estimate provides strong evidence for H1, by indicating that the average firm earns 1.03% of unexplained return over an 11-day period. In the fourth event period, the average CAR for a 7-day (-3, +3) event period amounts to 1.57%, which provides the strongest support for H1. More formally, the Patell Z-statistic of 2.398 represents a p-value 0.0082, which indicates statistical support at the 1% level. Finally, the last estimation window solely incorporates the actual event day with an average cumulative abnormal return of 0.56%. This respective mean CAR leads to a Patell-Z score of 0.348 with a p-value of 0.03639, which indicates a positive but non-significant return estimate.
Next, this paper focuses on the non-parametric analyses, which indicate comparable results and support for H1 but further include the greater estimation periods. Similar to the parametric tests, the research study applies two non-parametric tests to confirm the robustness of the results. As the results of the generalized sign test represent the main differences relative to the parametric tests, the focus of this section will be on the Generalized Sign Test.
The key difference to the parametric test lies in the significance of the longer event window periods. Firstly, the longest event window (-15, +15) incorporates 34 positive, and 29 negative unexplained return estimations. Based on these estimates, the resulting Z-score amounts to 1.665 and the p-value to 0.0479, which provides significant evidence to reject the null hypothesis of H1 at the 5% level. The subsequent 21-day estimation window (-10, +10) provides similar results with 33 positive abnormal returns and 30 negative abnormal returns. Consequently, the generalized sign z-value equals 1.452, which provides support for the hypothesis H1 at the 10% significance level (p-value = 0.073). Further, the 11-day event period (-5, +5) provides the greatest statistical support for H1 with 35 positive and 28 negative abnormal return estimates. The subsequent z-statistic corresponds to 1.971 with a p-value of 0.0243. This provides statistical evidence at the 5% significance level. Lastly, the two remaining event windows of 1 (-0, +0) and 7 (-3, +3) both experience 32 positive, and 31 negative unexplained returns, respectively. As a result, the Z-statistic follows the positive sign prediction but indicates non-significance for both event windows (Z-Statistic=0.437, p-value=0.331; Z-Statistic=0.59, p-value=0.2775).
To summarize, the results for various event timeframes (7-day, 11-day, 21-day, 31-day event windows), in both parametric and non-parametric estimation technique, indicate that organizations can derive economic value from the development of human capital.
Results of Regression Analyses
Following the event study description, the research study applies various regression analyses aiming to explain the variation of the previously identified abnormal returns. The abnormal returns from the event study will be used as the dependent variable in the following models. More specifically, the results indicate which externally available information, in form of performance ratios, enables stakeholders to explain the abnormal earnings possibilities related to investments in the development of human capital. These performance indicators (H2a-H2e) will all be tested for three different event period lengths. Despite the five possible event windows, I will focus on only event windows 3-5 (11-Days, 7-Days and 1-Day) due to the consistent use of these event window lengths in the accounting and finance literature (Brown and Warner, 1985). As mentioned before, the longer event windows are primarily to account for potential leakage and deferred trading activities. Generally, the regression results provide statistically significant evidence for various performance ratios. More specifically, the Research & Development intensity, Salary competitiveness and the PPE intensity explain part of the variation, resulting from the abnormal returns following human capital development. A more comprehensive analysis of the findings and associated hypothesis follows in the subsequent section.
Hypothesis 2a
The estimates for R&D intensity for estimation window 4 and 5 (-3, +3; -0, +0) provide no statistical support for the explanation of the abnormal returns (See Appendix II.C – Table 15 and 16). However, the 11-day event period provides statistical evidence in model 2 and 6 with the coefficients of -6.26 at a 10% significance level (Table 17). Despite the opposing sign relative to the prediction, this finding indicates partial support for Hypothesis H2a.
Hypothesis 2b
Similarly, to the previous hypothesis, the coefficients for the shorter event periods indicate non-significance for the salary competitiveness variable (Table 15 and 16). However, the estimates of -9.45 (Model 3) and -7.71 (Model 7) for the salary competitiveness variable deliver statically significant results in model 3 and 7 at the 5% and 10% significance level, respectively (Table 17). Nonetheless, the signs of the coefficients contradict the respective prediction.
Hypothesis 2c
In contrast to the previous hypothesis, the marketing intensity coefficient fails to provide significant coefficients in Model 1 and 3. Nonetheless, the estimates of 0.998 (Model 2) marginally supports the notion that marketing intensity (H2c) of an organization can explain a portion of the unexplained variation of the abnormal returns related to human capital investment. The coefficient is marginally significant at the 10% significance level.
Hypothesis 2d
The estimate for PPE intensity provides statically significant results in two of the three event periods (Table 15 and 17). More formally, the coefficients in the event window 1 and 3 provide marginal support, for H2d, at the 10% significance level across various model specifications. Notably, the respective coefficients for PPE intensity is negative across all models and event periods, which follows the previously non-specified direction of the hypothesis.
Hypothesis 2e
The results with respect to the interaction effect of physical capital and R&D intensity provide non-significant coefficients across all event period lengths and models. Despite the consistent sign predication, the interaction term delivers highly insignificant estimates. Moreover, this is also confirmed in Table 17 (Model 6), which indicates a significant effect of each individual variable on the abnormal returns relative to the effect of the interaction term. Conclusively, the results provide no significant support for H2e.
Results of Logit/Probit models
Descriptive statistics
First, an overview of the descriptive statistics is displayed in Appendix III.A. Table 18-19. Specifically, in Table 19, the comparison between guidance and non-guidance organizations indicates significantly greater scores in the key internal area, human capital, by organizations that provide earnings forecasts. However, the other internal design variables, namely decision-making and target-related practices seem to be similar across those two types of organizations. Further, the organizations that provide guidance also seem to be statistically different in other areas. More specifically, organizations that provide guidance seem to be larger, less risky, more profitable and to have greater analyst coverage relative to their non-guidance providing counterparts.
Hypothesis 3a
Table 20 reports the results of Hypothesis H3a, which are also in line with the aforementioned differences between guidance and non-guidance firms stated in the descriptive statistics. More formally, the coefficient of 0.018 (Management Score in Human Capital) indicates a positive influence of the internal human capital practices on the probability of issuing a management earnings forecast (p-value = 0,041). Furthermore, none of the remaining internal management practices has a significant effect on the likelihood of earnings forecast issuance. With respect to the control variables, the disagreement among analysts and return on assets seem to be key factors when managers decide whether they want to provide guidance to the capital market. The resulting coefficients provide evidence at the 5% and 10% significance level, respectively. As a result, the outcome in Table 20 supports the premise of Hypothesis H3a.
Hypothesis 3b
Firstly, the models include an additional variable to control for potential selection bias and non-randomness of the issuance of management forecasts. More specifically, the inclusion of the inverse mills ratio aims to account for the choice of providing a management forecast (Heckman, 1976). In other words, controlling for the correlation between error terms is specifically important in this setting, as human capital practice scores are expected to affect both the probability of issuance and the forecast properties of the management guidance (Lennox, Francis and Wang, 2011). The latter effect is also expected to be conditional on the actual issuance of a guidance forecast. Following this model specification, the forecast bias results, in both absolute and signed version, are displayed in Table 21, Column (1) and (2). The coefficients on the main independent variable of interest (Management Score in Human Capital) support the notion of inaccurate forecasts issuance by management at the 5% significance level. However, the sign opposes the predication and indicates a positive bias of the earnings guidance. With respect to the signed forecast bias, the internal target setting practices (Management Score in Target Setting) also show a significant positive effect on the directional bias of the management guidance (p = 0,0674). Further, numerous control variable coefficients also influence the forecast error and bias. Specifically, the length of the forecast horizon, potential loss-making and market-wide risk effects (Systematic Risk) seem to significantly bias the forecasts, at the 5%, 10% and 10% level, respectively.
Hypothesis 3c
The results in Table 21 – Column (3) follow the previous notion and suggest that firms with higher human capital practices scores (Management Score in Human capital) are more likely (less likely) to meet (miss) their own and analysts’ earnings targets. Furthermore, the internal target setting practice also increases the probability of firms reaching the EPS forecast set by themselves and their analysts. However, none of the other internal practices seem to significantly influence the probability of meeting EPS measures. With respect to the control variables, the variable for interanalyst uncertainty of the earnings forecast appears to significantly affect the likelihood of forecast fulfilment by the organizations.
Discussion of the Event Study
Hypothesis 1a
Both the parametric and non-parametric results of the event study indicate a clear and robust answer with respect to the first research question. These findings strengthen the premises of human capital development and its value creation potential. More specifically, the ATD BEST award receipt provides the external environment with information about the organization’s human capital development efforts. Following the semi-strong form efficiency assumption, the external market perceives this signal as previously unknown information, which then leads to the inclusion of future value opportunities into the current stock price and corporate valuation of the organization. In the following paragraphs, I will further discuss the individual performance indicators that enable internal and external stakeholders to explain this market reaction and identify potential differentiation strategies across companies.
Discussion of the Regression Results
Hypothesis 2a
As indicated by Model 2 and 6 in Table 17, the R&D intensity of organizations significantly affects the abnormal returns, resulting from a training award receipt. This does provide partial support for H2a; however, the findings oppose the predicted direction of this relationship. A potential explanation for these results may stem from the ambiguous reporting rules related to research and development. For instance, Lev, Sarath and Sougiannis (2005) investigate the extent and consequences of biases in R&D reporting. In their study, they find that reporting speed (conservative or aggressive) with respect to R&D expenses, as their bias of interest, significantly affects the resource allocation in the capital markets. More specifically, organizations strategically report R&D expenses, conservatively or aggressively, based on the difference between their R&D growth rate and profitability measures. As a result of this reporting bias, firms seem to be under- or overvalued, which manipulates the external parties and their allocation behavior. Similarly, this misreporting of R&D expenses or other complex theory implications may be responsible for the contradicting sign relative to the proposed hypothesis. To conclude, the significant findings indicate the need to further investigate into the R&D reporting process and potential future value expectations of investors with respect to R&D.
Hypothesis 2b
Similar to the previous hypothesis discussion, the coefficients for Salary competitiveness provide marginally significant support in model 3 and the complete model 7 (Table 17). Nonetheless, the signs of these coefficients do not follow the predicted direction set by H2b. A potential reasoning for this direction may be the preference of non-financial compensation relative to financial compensation. More specifically, modern compensation packages include flextime, telecommuting options or other non-financial perks, which are highly valued by individuals. This premise is also confirmed by Schlechter, Thompson and Bussin (2015), who examined the attractiveness of non-financial rewards for prospective employees in knowledge industries. By collecting questionnaire data about the perceived level of job attractiveness, they identify the importance of non-financial reward elements (e.g. career perspectives, training opportunities) on employees’ perceived attractiveness of potential job offerings. As a result, employees may assume that these opportunities are relatively modest in highly-demanding but highly-compensated jobs (high salary competitiveness ratio).
Hypothesis 2c
The third sub-hypothesis H2c focuses on the effect of Marketing Intensity on the abnormal returns. This effect is marginal significant (p-value = 0.070) in the fourth event window (-3, +3) and follows the predicted direction (Table 16). Thus, the advertising activities and potential utilization of “advertising-assets” seem to be interconnected with highly-skilled labor and the investments in this workforce. Nonetheless, the findings must be interpreted with caution due to the marginal support in only one model specification and event window length.
Hypothesis 2d
The event windows 3 and 5 (Table 15 and 17) both provide strong support for a direct effect of PPE intensity on the human capital induced abnormal returns. Furthermore, as indicated in the hypotheses section, one can argue for either a positive or negative effect on the dependent variable. However, the findings indicate a consistent negative relationship on the abnormal returns. As a result, the negative direction suggests that investors may interrelate high levels of physical capital with lower skill requirements for human capital. This argumentation would also be supported by the relatively high frequency of manufacturing firms in the sample. More specifically, the trend towards physical labor replacement in this industry remains relatively high, which would support the aforementioned relationship between physical capital and skill requirements for human capital. Despite the significance of this variable, additional tangible asset measures need to be examined to strengthen the assumptions with respect to automation and related human capital requirements.
Hypothesis 2e
Regarding the effect of R&D and physical capital, no model specification across all the event window provides significant support for this interaction variable. Consequently, the findings fail to provide supporting evidence for H2e; however, this also presents a compelling deviation from the previous results. More specifically, the individual effects of both R&D (H2a) and PPE (H2d) intensity provide significant evidence, while the interaction between the two measures yields insignificant results. Further, this result seems to indicate that the marginal influence of R&D intensity differs across various levels of PPE intensity, and vice versa. Nonetheless, this non-significance may also result from the difficulties with respect to adequate R&D reporting.
Discussion of the Logit/Probit Model Results
Hypothesis 3a
As previously mentioned, the positive coefficient on the human capital practices, depicted in Table 20, provides support for the expectation that human capital practice quality has a significant impact on the probability that management issues an earnings forecast. Consistent with Verrecchia (1990) and Penno (1997) findings, that the quality of private information positively influences the probability of forecast disclosures. However, it is important to highlight the nature of the disclosed information. The greater likelihood of disclosure suggested by Verrecchia (1990) only holds for proprietary information. The human capital practices of an organization fit into this information type and, therefore, fulfill the premise of the respective paper. On the other hand, Penno (1997), who builds on Dye (1985) and Jung (1988), shows that the common economic notion of higher-quality information and the accompaniment of greater voluntary disclosure fail in a non-proprietary setting. On the contrary, in such environments, the disclosure frequency is mostly independent of the information quality. Importantly, certain information quality thresholds even decrease the voluntary disclosure frequency. However, this premise only holds when the ex-ante information quality is increasing in the associated ex-post information quality (Penno, 1997). Such scenarios highlight the importance of identifying the correct private information type and its quality when intending to voluntarily disclose information to the external market. In addition, the non-significance of the other internal management practices specified in this model also provide key insights with respect to H3a. More specifically, the individual significant effect of human capital practice quality on the forecast issuance probability reduces the potential of other factors (e.g. overall internal design and management quality) to drive this relationship.
The direction of the significant control variables follows prior literature and expectation. For instance, the authors Cotter, Tuna and Wysocki (2006) discover that management guidance is more likely when analysts’ forecast dispersion is low. Intuitively, lower levels of uncertainty across analysts, with respect to earnings forecasts, are associated with more consensus about the future prospect of the firm. This consensus can be used by management to guide analysts to a common (beatable) target, by issuing an earnings forecast. Similarly, organizations with higher levels of operating performance (ROA) are inclined to keep this measure constant and may use the private information, and respective guidance, to signal this efficiency or further enhance the performance ratio.
Hypothesis 3b
With respect to the forecast properties, forecast accuracy and the associated bias of the forecast, the results confirm a statistically significant inaccuracy and bias; however, the bias seems to be optimistic. The former result opposes prior literature with respect to the incentive of providing highly accurate earnings forecasts. However, despite the rewards for high disclosure accuracy, management may focus on the subsequent performance implications (e.g. meeting earnings targets, earnings surprise), which incentivizes the provision of more inaccurate and pessimistically biased guidance reports. Following the significant forecast error in Column (1), the next section will focus on the findings regarding the optimistic forecast bias. More specifically, Table 21 – Column (2) indicates that human capital practice quality (Management Score in Human capital) and target setting quality (Management Score in Target Setting) positively bias the earnings forecast issued by management. This result opposes the predicted expectation of managerial incentives to create more achievable (beatable) targets and subsequently, potential positive earnings surprise, following the achievement of these targets. Importantly, this bias is also supported by the forecast inaccuracy displayed in Column (1), where human capital practice quality causes a significant increase in the forecast error. However, the main focus relates to the directional bias of the earnings forecast by management.
A potential explanation for the over-optimistic estimation, with respect to the EPS measure, may result from overconfidence in the forecast ability of the management team. This overconfidence may result from the high quality of human capital and the related private information of human capital practices possessed by management. For instance, Hribar and Yang (2016) find overconfident managers issue earnings forecast more frequently, positively bias these forecasts and provide less precise forecasts. However, their results also indicate that these overconfident and over-optimistic managers are more likely to miss both analysts’ as well as their own targets. This subsequent premise does not hold in this research study, as previously described in the result section of hypothesis H3c. As a result, the optimistic forecast bias may be a signal of potential future value creation, resulting from the high levels of human capital and related practices. Similarly, the internal target setting practice quality leads to positive and significant biases of the earnings forecast. The explanation follows a similar argumentation; managers, who are confident in their target setting abilities may assess future earnings in a highly positive way. Alternatively, the high quality of internal target setting (e.g. balanced mix of financial and non-financial incentives, clear communication of incentives and weights, etc.) may incentivize employees to increase efficiency or aspire additional growth, which enables the firm to accomplish these highly optimistic earnings targets. Lastly, the significant control variables Systematic Risk, Loss, Previous Award and Horizon follow the direction of prior literature. For example, greater exposure to market-wide effects and/or the resulting riskiness leads to more uncontrollable events, which reduce forecast accuracy and, subsequently, bias the forecasts. Similarly, a longer Horizon (e.g. greater difference between management forecast date and fiscal year-end date) suggests a greater forecast error and directional forecast bias (Ajinkya, Bhojraj and Sengupta, 2005).
To summarize, the results do support the managerial incentive to provide less accurate forecasts, however, the predicted directional bias contradicts the argumentation of more achievable (beatable) EPS targets. Overall, the results of the analysis of hypothesis H3b are not consistent with the predictions made in this paper.
Hypothesis 3c
The last hypothesis (H3c) focuses on the probability of fulfillment of the earnings targets estimated by the management and the following analysts. In Table 21 – Column (3), the findings clearly indicate that the quality of human capital practices within the organization have a significant effect on the probability of meeting/beating the earnings targets. Furthermore, the internal target coverage practice quality also provides a significant increase to the likelihood of achieving the respective EPS targets. These results follow the argumentation in the hypothesis development and lead to the rejection of the null hypothesis. Interestingly, this result, in combination with the findings in Column (1) and (2), indicates that managers just meet the respective targets; however, management makes the achievement of these forecasts more difficult through the positive directional bias. The former statement with respect to meeting/beating the EPS targets can be inferred from the positive effect of human capital practice quality on forecast errors. However, the latter result enhances the strength of this hypothesis. Not only does the organization avoid potential earnings surprises with the fulfillment of the EPS targets, but also enables an initial signaling of future value creation with the initial over-optimistic forecast. Among the control variables, only the dispersion among analysts significantly influences the probability of meeting the management and analyst targets. Similar to the previous paragraph, higher analyst disagreement leads to more uncertainty with respect to the future prospects of the firm, which will result in the provision of less achievable target by analysts. To conclude, the evidence supports that organizations with higher human capital practice quality are more likely to achieve their earnings targets.
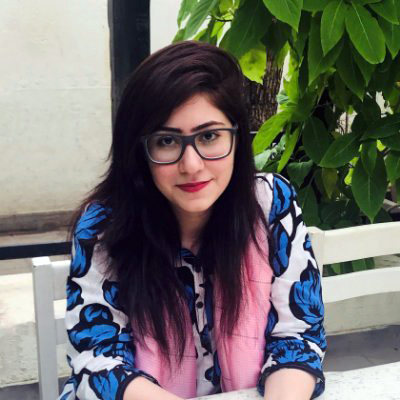
Freelance Writer
I’m a freelance writer with a bachelor’s degree in Journalism from Boston University. My work has been featured in publications like the L.A. Times, U.S. News and World Report, Farther Finance, Teen Vogue, Grammarly, The Startup, Mashable, Insider, Forbes, Writer (formerly Qordoba), MarketWatch, CNBC, and USA Today, among others.