- StudySolver
- /Chapter 3: Cfd Simulations
Chapter 3: Cfd Simulations
Chapter 3: CFD Simulations
In this chapter, the simulation systems adopted in this proposal is presented, mesh configuration, the geometry of the computational domain, materials that have been employed in the simulation are described.
CFD Simulations
CFD simulations were completed to gain additional insight into the focusing effect of the orifice and potentially make improvements to its geometry. The ideal orifice would focus only particles within a narrow size range. In addition, all particles within the size range would be focused regardless of their position upstream. It follows that particles outside the size range would experience large deflections from the centerline immediately after the orifice.
Middha and Wexler (2003) [1] studied precisely this effect, where nanoparticle-laden choked flow through an orifice was simulated using CFD. This proposal attempts to reproduce the results of Figure 3-15 from Middha and Wexler (2003) [1]. The model will be used as a baseline for future studies improving the performance of nanoparticle focusing on the aerodynamic lens.
The Geometry of Computational Domain
The geometry and dimensions of the domain are shown in Figure 3-1. For making this work more realistic, the orifice diameter was 3 mm and 1.6 mm thick. A length of 50 mm before the orifice and 60 mm after the orifice was simulated, and the inner diameter of the tube was 35 mm, which is one of the necessary configurations in the industry, is assumed. It is important to note that computational fluid dynamics (CFD) is only valid in three dimensions (3D) and two dimensional (2D) axisymmetric geometries. Hence, by employing 2D axisymmetric modeling, CFD modeling it can be used.
Figure 3 1: Geometry of Computational Cell
Dotted lines in the Figure 3-2 below show how the domain was divided into three areas of different mesh densities, which are labeled. The model was simulated as a 2D axisymmetric problem.
Figure 3 2: Geometry and dimensions of the CFD model (mm) divided into three areas of different mesh densities
Mesh Configuration
A screenshot of the model mesh is shown in Figure 3-3. Referring to Figure 3-2, elements in area 1 were set to 0.6*0.6 mm, while area 2 elements were arranged to 0.2*0.2 mm due to flow acceleration in the x and y directions. Lastly, area 3 elements were 0.5*0.7 mm with a bias factor of 5 in the vertical direction due to high-velocity gradients in the y-direction. Face meshing was applied to the entire mesh to obtain elements with less skewness. The maximum orthogonal skewness and aspect ratio was 0.4 and 4, respectively. In total, there were 31104 elements in the domain.
Figure 3 3: Mesh Screenshot model of base case
One of the principal purposes of doing this simulation is trying to capture and understand the near wall behavior of nanoparticles, the velocity component perpendicular to the pipe surface, and drag coefficient at the vicinity of the pipe wall. Consequently, the best decision or a comparatively fine mesh in the proximal wall part, or in the boundary layer is needed. However, a small computational effort required that the mesh should not be too fine. As a compromise, a non-uniform quadratic mesh, as illustrated in Figure 3-4, is used. In Figure 3-4, the lower edge corresponds to the axis, and the upper edge corresponds to the pipe wall.
From the Figure 3-4, we can detect that there is a finer mesh at the near-wall region and a comparatively fine mesh in the area near the centerline. However, we can notice that the mesh at the bottom is part of the pipe mesh, wall, and axis are labeled in the Figure 3-4; the one below is a drawing of partial enlargement of the region encompassed by the red box in the lower image.
Figure 3 4: Mesh Configuration and Part of the Mesh of Computational Cell
This magnified image is used to give a visual idea of the non-uniform mesh. The two-way arrow on the right side of the mesh is indicating that the mesh becomes finer near the wall, but gradually become fine when moving toward the centerline. Another reason for using fewer elements along the axis is that changes of flow properties along the axis are expected to be more gradual, so it is unnecessary to employ a finer mesh or non-uniform mesh along the axis direction. Instead, much fine, the uniform mesh is used along the axis direction.
As it is mentioned above, by using the idea of axisymmetric modeling, the computational cell can be simplified to a rectangle. 50 uniform divisions in the axis direction and 50 non-uniform divisions in the radius direction are made. Hence, in total, there are 31104 elements and 30,000 nodes. The mesh size surrounding the particle is too fine to predict the variation of velocity, pressure, and temperature with high accuracy, however, it has normal size near the walls to increase the calculation speed (Figure 3-3). In Figure 3-3 the mesh is generated with 31104 mesh elements; therefore, the nanoparticle has a diameter 25 nm including the possible adsorption layer [35] which is the actual regime for mutual interaction between nanoparticle surface and air molecules.
Solution Setup
Details of ANSYS Fluent settings for solving the flow are assumed. The flow of the carrier gas was considered as a transient state, compressible, laminar, viscous flow, and axisymmetric, also we used the solver type by Pressure-Based. Moreover, boundary conditions were considered by assuming the inlet pressure 2700 Pa with 35 mm hydraulic diameter, 5% backflow intensity, 35 mm backflow hydraulic diameter for outlet pressure, also for the walls we assumed there is no slip and 0.5 roughness constant. The flow solution was verified by ensuring all residuals were less than 〖10〗^(-5) , the average outlet velocity and mass flowrates were constant. A grid independence study was also performed by repeating the simulation with a finer mesh. However, all element dimensions of the original mesh were reduced by 20%, resulting in 31104 elements.
By using the surface injection type, particle diameters from 1 to 25 nm were released at the inlet at various radial positions. The particle material was chosen as Carbon and particle type in this simulation was Inert, and this selection only determines the density used in calculations. The pre-defined drag law models in Fluent did not take into account the Cunningham slip correction factor for submicron sized particles, so a user-defined function (UDF) was used instead as shown in Appendix A. The next step is running to calculate our mesh file into Fluent 16.2 and setting up the model which we plan to use in conjunction with the multiphase by using Eulerian-Eulerian Model. So, we need to enable the Eulerian-Eulerian Model and make the volume fraction parameters to be Implicit for the formulation. The maximum number of the steps in the tracking parameters was 500 with 5 length factors. For different injection size of the particles in the inlet, a convenient representation of the droplet size distribution was the Rosin-Rammler expression. Figure 3-5 below shows the chart of the Multiphase Model used in the Eulerian-Eulerian Model for the solution method in ANSYS Fluent:
Figure 3 5: Solution Method in ANSYS Fluent Chart of Multiphase Model Used in Eulerian- Eulerian Model
We have to define the physical properties of the unburnt material in the domain; therefore, we choose a spherical drag law in the physical models and standard parcel release method. Hence, we select the particles size in three stages, (1-10 nm),(10-25 nm) and (25-100 nm) to show the effect of nanoparticle interaction with the wall in fluid flow (Figure 3-6).
Figure 3 6: Three stages of the particles size, (1-10 nm), (10-25 nm) and (25-100 nm)
In section 3.3 below, we explained the properties of the material for the fluid, particles, pipe, and orifice. The particle was assumed to be spherical and zero for the gravity. Also, simulations were done for a range of particle diameters with an upstream temperature of 298 K and source pressure of 2700 Pa. Moreover, the particle injection points were varied to examine the dependence of focusing on the initial position. After run calculation for our model, we obtained the following results that show us the results for four different times as shown in Figures 3-7 and 3-8:
(b)
Figure 3 7: The particle traces colored by particle residuals at (a) 2.5e-02 second, (b) at 6.5e-02 second
(b)
Figure 3 8: The particle traces colored by particle residuals (a) at 1.2e-01 second, (b) at 1.23e-01 second
Multiphase Particle Modeling
Modeling Multiphase Flows
In this section, some parts of the general theoretical background of multiphase flow simulation are presented. The multiphase flow is quite a general term and besides the gas-solid flows. Despite the similar overall setting, the exact models and computational techniques can differ quite a lot from case to case. In gas-solid flow simulation, there are two basic approaches how to model the solid particles: the Eulerian-Eulerian approach and the Eulerian-Lagrangian approach. In the former of these two, the solid particles are considered as a secondary phase, and they are modeled with equations similar to the classical Navier-Stokes flow equations. The Eulerian approach is currently commonly used in multiphase simulations, and it is a relatively fast and usable approach. It has been implemented in commercial CFD ANSYS software, and it is also the method of choice in this proposal.
This section discusses the general multiphase models that are available in ANSYS Fluent. In solving any multiphase problem, the first step is to determine which of the regimes described in this section best represents our flow. This section provides some broad guidelines for determining appropriate models for each regime, and also it gives details and how to assess the degree of interphase coupling for flows involving particles, and the proper model for different amounts of coupling. The multiphase flow equations consist of a continuity and momentum equation for each phase. The continuity and the momentum equations for gas g and particle solids p can be written as follows:
∂/∂t (α_g ρ_g V_g )+∇ .(α_g ρ_g V_g V_g )=-α_g ∇p+∇ .τ_g+α_g ρ_g g+K_gp (V_p-V_g) (3.1)
∂/∂t (α_p ρ_p V_p )+∇ .(α_p ρ_p V_p V_p )=-α_p ∇p-∇p_p+∇ .τ_p+α_p ρ_p g+K_gp (V_g-V_p) (3.2)
Where p_g the gas phase pressure, p_p the solid phase pressure, K_gp the inter-phase drag coefficient and ρ_g and ρ_pare the gas and solid densities. The Wen & Yu model [101] is based on a wide range of observations, and it is considered to have better accuracy in dilute flows. For Wen & Yu model is:
K_gp=K_WY=3/4 C_D (〖α_p α〗_g ρ_g |V_g-V_p |)/d_p α_g^(-2.65) (3.3)
Where the coefficient C_D comes from:
C_D={█(24/〖Re〗_p [1+0.15(〖Re〗_p )^0.687 ] 〖Re〗_p≤ [email protected] (Figure 3.9) 〖Re〗_p>1000)┤ (3.4)
Figure 3 9: Drag coefficient of a particle
In this context Reynold’s number appearing in the correlation can be written as:
〖Re〗_p=(α_g ρ_g |V_g-V_p |d_p)/μ_g (3.5)
Eulerian-Eulerian Model
Currently, the typical approach to the model aerodynamic lens is the Eulerian-Eulerian model which is also known as a multiphase model. In this approach, both the gas phase and the solid phase are modeled as continuums. The model differs by their treatment the second particle phase. However, the Eulerian-Eulerian model treats the particle phase as a second continuum phase calculated from mass conservation principles [94]. In this case, the solution is typically interpreted regarding a concentration field, since individual particles cannot be tracked in this method. The Eulerian-Eulerian model in that it treats the second phase as a continuum collection of individual particles. The primary forces considered are drag force, Brownian force, gravitational force, and buoyancy force [95].
Though this is not a complete list of forces, cumulatively, they comprise a high majority of forces which can affect particle trajectories. The resultant force is then calculated at continuum time intervals, and the particle is advanced according to the force. We can include a continuum phase in our ANSYS Fluent model by defining the initial position, velocity, pressure, size, and temperature of individual particles. These initial conditions, along with our inputs representing the physical properties of the continuum phase, are utilized to initiate trajectory calculations. The procedure to set up and solve a problem involving a continuous phase is described below (Figure 3-10). Only the steps explicitly related to continuum phase modeling are shown here. The six steps in each iteration are illustrated below in Figure 3-10:
Figure 3 10: Flow Chart of Pressure-based Used in ANSYS Fluent
The momentum equation of the nanoparticles includes several forces such as drag force F ⃗_Dr, gravitational force F ⃗_Gr, Brownian motion force F ⃗_Br, and buoyancy force F ⃗_buo. The governing equations for the spherical-solid nanoparticles are written as follows:
m_p 〖d ⃗u〗_p/dt= F ⃗_Dr + F ⃗_Gr +F ⃗_Br + F ⃗_buo (3.6)
Where, u ⃗_P= 〖d ⃗r〗_p/dt, the term r_(p )is the location we are interested in and where the nanoparticle’s centers are assigned. The drag force that nanoparticles experience from the gas phase is calculated by applying the Stokes drag law as follows:
F ⃗_Dr= 3 π d_(p ) μ (u ⃗_g- u ⃗_P) (3.7)
Here, u ⃗_g is the gas phase velocity where the nanoparticle is located. The gravitational and buoyancy forces experienced by the nanoparticles are obtained from the upcoming expressions, which is presented in equations (3.6) and (3.7): F ⃗_Gr =(π d_(p )^3 ρ_p)/6 g ⃗, (3.8)
F ⃗_buo =(π d_(p )^3 ρ_g)/6 g ⃗, (3.9)
Finally, the continuous collisions between a single nanoparticle and the surrounding molecules of the carrier phase correspond to the stochastic displacements. The Brownian force reflects this rapidly oscillating behavior of the nanoparticle. The present numerical work employed the proposed formula for the Brownian force and is as follows:
F ⃗_Br= ξ√((〖6 π μ d〗_(p ) K_(B ) T)/(Δt Cc)) (3.10)
The term T demonstrates the isothermal medium temperature, which is equal to 298 K, ξ is called the Gaussian random number with zero-mean unit-variance-independent, K_(B ) is the Boltzmann constant (1.38×〖10〗^(-23) J/K), and Δt is the simulation time step. The following relation is employed to evaluate the dimensionless Cunningham correction factor:
Cc = 1+2kn (1.257 + 0.4e^(-0.55kn)) (3.11)
It should be noted that since the Knudsen number is much less than unity, the Cunningham correction factor Cc is nearly equal to unity. It is worth mentioning that Brownian force could be rewritten with respect to Brownian diffusion coefficient, D_B, as follows:
D_B=(K_(B ) T)/〖3 π μ d〗_(p )
F ⃗_Br= ξK_(B ) T√(2/(Δt D_B )) (3.12)
The effects of the gravitational field and Brownian motion have been investigated, and the velocity distribution of nanoparticles and their relative concentration will be presented in the proposal.
The Rosin-Rammler Diameter Distribution Method (RRDM)
Regarding particle size distribution in ANSYS Fluent, the only available functions are Rosin Rammler Distribution Method (RRDM) and Uniform distribution. Because we have different sizes of particles, we have to use the Rosin-Rammler Distribution Method (RRDM). We considered that the size of nanoparticles is sub 25 nm (sub 2.5000e-8 m).
Base Fluid, Nanoparticles, Orifice, and Pipe
In this current section, the properties of the base fluid, nanoparticles and the pipe utilized in this simulation are discussed. Moreover, the base fluid used in the simulation is air, and its properties are listed in Table 3-1, while the nanoparticles used in the simulation are spherical Carbon particles with a diameter of 1-25 nm, and its properties are shown in Table 3-2. Also, both of the pipe and orifice are made of stainless steel, and its properties are shown in Table 3-3.
Table 3 1: Properties of air
Base Fluid Density (Kg/m3) Viscosity (Kg/(m.s)) Specific Heat (J/(Kg.K)) Thermal Conductivity (W/m.K)
Air 1.225 1.81×10−5 1000 0.024
Table 3 2: Property of nanoparticle (Carbon)
Nanoparticle Density (Kg/m3) Viscosity (Kg/(m.s)) Thermal Conductivity (W/m.K)
Carbon 2250 1.789 ×10−5 0.33
Table 3 3: Property of pipe Wall and orifice
Pipe & Orifice Wall material Density (Kg/m3) Specific Heat (J/(Kg.K)) Thermal Conductivity (W/m.K)
Stainless-Steel 7700 910 16.3
Solution Strategy
Nanoparticle inlets are essentially axisymmetric assemblies of orifice or nozzle. The sharp plate orifice is a limiting case of the nozzle; however, in a typical nanoparticle inlet, an orifice or a nozzle is utilized to accelerate the particles to sonic speeds, (Figure 3-11).
Figure 3 11: The Flow Velocity in the Orifice
Sharp plate orifice is more efficient in focusing small particles as it produces maximum acceleration and focuses hence the smallest particles efficiently. Passing nanoparticles through a sharp orifice where the flow is choked focuses a narrow range of particle sizes. This principle was employed to verify the focusing mechanism in a sharp orifice. However, to validate the theory above, a CFD by using ANSYS code Fluent (16.2) was utilized to simulate the fluid flow and particle movement in an orifice as shown in Figure 3-12.
Figure 3 12: The particle motion of an orifice
A 3 mm axisymmetric orifice with 1.6 mm thick was used since simulation data is available for this configuration. The grid was generated using geometry, a preprocessor tool provided by Fluent. Moreover, the flow domain was divided into mappable subdomains, and a structured quadrilateral mesh was generated for the flow domain. Therefore, the domain meshed such that the skewness was below 0.4 in the entire domain and the aspect ratio was below 4. The mesh was made denser near the orifice to resolve any large gradients in velocities and was rendered fine in the regions with small velocity gradients. Computations were also carried out for a much finer mesh to establish the grid independence of the results, as shown in Figure 3-3 in section 3.1.2.
Validate the Model
A Figure 3-13 below shows a typical particle trajectory which crosses through an orifice, where X = 0 corresponds to the upstream face of the orifice plate. The particles start (50 mm) upstream of the orifice and follow the streamlines until the particles approach the orifice. At the orifice, the particles come very close to the axis and then move radially away from the axis as the particles progress downstream from the orifice.
Figure 3 13: the particles come very close to the axis
Moreover, the particle trajectories are followed up to a distance of 60 mm downstream of the orifice exit. A unique focal point is seen to exist for some particle sizes [2]. However, the particles from the off-axis region do not necessarily pass through that point. Also, the optimally focused particle diameter corresponds to a Stokes number Stk* ∼ 1, which is again in accordance with computational observations.
The focusing of the particles was found to be independent of downstream pressure as foreseeable, provided that the downstream pressure is low enough to ensure choking flow conditions. It was observed that the orifice focused on a narrow range of particle diameters. It can be seen from Figure 3-14 that the range of particles focused is a function of the radial distance and reduces the radial distance from the axis. Table 3-4 summarizes these results for upstream pressure.
Table 3 4: Summary of results for 3 mm orifice at an upstream pressure
Pressure (Pa) 〖Stk〗_f σ_g
2700 1.0 1.07
Analysis of the model showed that focusing also depends on the initial position of the particles upstream. Also, a wide range of nanoparticle sizes would be focused into the small orifice of 3 mm diameter. The pressure gauge was also installed before and after the orifice. These aspects should be addressed to improve the performance of the aerodynamic particles focusing. As a result, Figure (3-18) shows us the upstream and downstream pressures in the aerodynamic focusing versus orifice size selected.
Figure 3 15: The work of Middha and Wexler
Figure 3 16: The axisymmetric geometry of the orifice Figure 3 17: The axisymmetric orifice by Middha and Wexler
Figure 3 18: The pressures in the aerodynamic focusing versus orifice size
From the agreement between these numerical findings, we conclude that the model simulates the flow field accurately, so we can use it to design aerodynamic lens. CFD simulations were completed to investigate parameters an affecting particle focusing characteristics of an orifice. Therefore, the base simulation in the work of Middha and Wexler (2003) [1] was replicated and results in agreed well as shown in Figure 3-14 above. Also, a comparison of simulation results, the work of Middha and Wexler (2003) and the Experimental result is shown below in Figure 3-19.
Some differences can be seen with particle sizes in this Figure. However, in Figure 3-19 the simulation results matched well with the experimental results but there are some differences can be seen with the work of Middha and Wexler (2003). The discrepancy can be attributed to use the aerodynamic lens which consisting of one sharp orifice instead of the conventional flat orifice.
Figure 3 19: Comparison of results from the simulation using ANSYS and work of Middha and Wexler (2003) with the experimental
Although a solution to achieve choked flow was to reduce the diameter of the orifice hole, the remedy would result in fewer particles entering the orifice. Considering that nanoparticles were charged, a response was still obtained, which indicates that the ANSYS Fluent has a sufficient limit of detection for the application. Precise measurements were observed throughout the 30,000 nodes, verifying the ability of the orifice to detect nanoparticles.
Chapter 4: Results and Discussions
Case 1: Laminar Fluid Flow with 4-10 nm Nanoparticles
In this case, we measured nanoparticles in the inlet of the pipe with air, the range size of particles was between 4-10 nm sizes of the particle. The particles move through the fluid in ?-direction and has very small periodic motion in ?-direction. Therefore, the boundary and initial conditions were temperature 298 K, pressure (inlet nanofluid: 2700 Pa, initial nanoparticle: 0.001 m/s). Figure 4-1 and Table (4-1) show the typical simulated of the particle traces 4-10 nm in the flow tube colored by particle residuals which move close to the axis after the first stage of modeling.
Figure 4 1: The particle traces 4- 10 nm gradient expected in the flow tube colored by particle residuals at 2.7e-01 second
Table 4 1: Laminar Fluid Flow with 4-10 nm Nanoparticles
Case 2: Laminar Fluid Flow with 10-25 nm Nanoparticles
In the second case, to show the effect of nanoparticle interaction with the centerline in fluid flow, firstly, the model was performing with size nanoparticle 10-25 nm; then, the nanoparticles interaction effect was studied. As a result, Table (4-2) illustrates the particle traces 10-25 nm gradient expected in the flow tube colored by particle residuals. According to the particle traces counter the parameters gradient is high in the entrance orifice and not fully developed as shown in Figure 4-2 below.
Figure 4 2: The particle traces 10-25 nm gradient expected in the flow tube colored by particle residuals at 2.7e-01 second
Table 4 2: Laminar Fluid Flow with 10-25 nm Nanoparticles
Case 3: Laminar Fluid Flow with Many Adjacent Nanoparticles 25- 100 nm
In this case, the effect of many adjacent nanoparticles 25-100 nm, instead of 4- 25 nm nanoparticles were studied. The initial conditions and the boundary are the same as case 1. Moreover, Figure 4-3 illustrates the particle traces 25-100 nm gradient expected in the flow tube colored by particle residuals and Table (4-3) shows nanoparticles size which used in this case.
Figure 4 3: The particle traces 25- 100 nm gradient expected in the flow tube colored by particle residuals at 2.7e-01 second
Table 4 3: Laminar Fluid Flow with Many Adjacent Nanoparticles 25- 100 nm
Compared with the results and gradient expected in the flow tube related to the model of Figures 4-2 and 4-3, it is clear that the nanoparticles cause an increase in distribution from the axis to the fluid during the orifice which is shown by the particle tracking of these two Figures. However, this improvement is due to the size effects of the nanoparticles. The model of many adjacent nanoparticles (case 3) clarifies how the impact of nanoparticle interaction with the centerline in fluid flow. Also, the modeling of two cases (1 and 2) clarifies the nanoparticle size effects on the nanoparticle interaction with the centerline in fluid flow. Figure 4-4 illustrates the scaled residuals.
Figure 4 4: scaled residuals (Time = 2.7e-01)
As seen in Figure 4-4, residual plots show the residual values have reached the specified tolerance. After the simulation, we note residuals have decreased by at least three orders of magnitude to at least 〖10〗^(-3). For the segregated solver, the scaled velocity residual decreased to 〖10〗^(-5). Also, the scaled species residual decreased to 〖10〗^(-4) to achieve species balance. Therefore, it can imply the effect of more particles in the fluid base that researchers have mentioned [45, 46].
The grid has approximately 30,000 nodes, and the converged solution does not change significantly when the number of nodes is quadrupled. Convergence of the solution is achieved within 1000 iterations using the grid and numerical scheme described above. Here, the convergence criterion is satisfied when the total sum of the mass residuals for the control volumes falls below the tolerance value of 〖10〗^(-10) kg/s, which is approximately 〖10〗^(-4) percent of the total droplet inflow. Computational simulations showed that penetrations of 4-40 nm particles range from 35% to 80%, and all particles are focused to some extent. Moreover, Computational simulations showed that the possibility to focus and measure particles larger than 40 nm but the inability to focus or measure particles smaller than 4 nm as shown in Figure (4-5) and Table (4-1):
Table 4 4: Computational simulation result
Figure 4 5: Focus particles larger than 40 nm
When many nanoparticles are close to each other, the influence on the area will be more than more due to the hydrodynamic interaction. It, in turn, increases the distribution of the nanofluid at macro scale for large particle because small particles are faster than large particles. The contours of velocity magnitude in the flow are shown in Figure (4-6 a, b).
(a)
(b)
Figure 4 6 (a, b): Contours of velocity magnitude in the flow.
As expected, subsonic flow occurs before the orifice and becomes supersonic afterward, Figure 4-7.
Figure 4 7: Flow Velocity before and after the orifice
Pathlines of particles injected at the inlet are shown in Figure 4-8, and the colors represent velocity. However, a focusing effect can be seen as more particles are closer to the axis after the orifice.
Figure 4 8: Pathlines of injected nanoparticles showing velocity
Results were found to best match those of Middha and Wexler (2003) when a 3.3 µm gas mean free path was used. This value corresponded to a pressure of 2,050 Pa as shown in Figure 4-9, which suggests λ should be calculated at the orifice rather than before it. The radial position of nanoparticles at the outlet was obtained from Fluent. The Stokes number corresponding to every particle size simulated was calculated using equation (4.1): Stk=(τu_o)/d_c =( ρ_p d_p^2 C_c u_0)/(18 μd_f ) (4.1)
The values of constants ρ_p, u_o, μ, and d_f were 2,250 kg/m^3,340.39 m/s,1.789*〖10〗^(-5) kg/m.s, and 3 mm, respectively.
Figure 4 9: Mean static pressure (Mixture).
The Cunningham slip was calculated with the following correlation (Middha and Wexler, 2003) [1]:
C_c=1+K_n [1.257+0.4e^((-1.1/K_n ) ) ] (4.2)
As particles pass the orifice, the gas mean free path decreases. According to the comparison of Figures 3-13 and 3-14 results in chapter 3, each plot is denoted by Rx, where x is the initial radial position of the particle upstream in mm. The simulation results matched well with the work of Middha and Wexler (2003) [1]. Although the base orifice design produces a focusing effect, it is likely not optimal for measuring particle size distribution. Particles are focused in a small area as seen by the minimum point of each plot in Figures 3-13 and 3-14. Particles focused by the orifice also depends on their initial location upstream, which is undesirable. This can be seen by the minimum point of each plot in Figures 3-13 and 3-14 shifting to the left as Rx increases.
Comparisons Between The CFD Simulation and The Experimental Data
Comparisons are made between the CFD simulation of the gas-solid (air-particles) flow and the experimental data obtained using the DMA technique.
Axial Velocity
Figures (4-10) and (4-11) show the comparison between the mean axial velocity profiles obtained by the CFD simulation and the DMA measurements. As shown in Figure (4-10 (a)), the measured and experimental axial velocity profiles show a downward flow region at all x-axis coordinates. In the same manner, Figures (4-10 (b, c, and f)) show that the predicted axial velocity profiles are mainly of a downward flow region at all x-axis coordinates. However, the measured axial velocity profiles indicate an upward flow pattern at the center of the pipe and a downward flow pattern at the wall. The predicted and measured axial velocity profiles are shown in Figure (4-10 (d, e)), indicating the presence of upward and downward flow regions at the center of the pipe and close to the wall respectively. Therefore, from Figure (4-10 (b, c, and f)) the computational model fails to predict the upward flow as observed in the measured axial velocity profiles.
Figure 4 10: Comparison of the CFD Results and Experimental Data for Mean Axial
This disagreement is due to the effect of natural rubber bungs used in experimental measurements to specify the split ratio as a function of mass flow rate passing through each outlet. The flow direction is expected to have changed as the flow reaches the bung at the outlet. The axial velocity profiles presented in Figure (4-10 (a- f)) show that the simulation results are under-predicted when compared with the experimental data. For example, Figure (4-10 (d)) indicates that the maximum upward axial velocity for the experimental data is 0.08 m/s, but in the simulation result, it is 0.045 m/s.
Figure 4 11: Comparison of the CFD Results and Experimental Data for Mean Axial
The best correlation between the experimental measurements and the predicted results in Figures (4-10 (a-c)) was obtained close to the wall region at negative values of the x-axis. Here, an agreement was within 5-10%. The same trend is achieved as we move closer to the wall at positive values of the x-axis, as shown in Figure (4-10 (d-e)). The predicted and measured axial velocity profiles as shown in Figure (4- 11 (a-e)) indicate the presence of upward and downward flow regions at the center of the tube and the wall respectively.
As shown in figure (4- 11 (a and b)), the predicted axial velocity profile is found to be greater than the experimental data at the center of the tube. However, as we move closer to the wall, the simulation results are found to under-predict when compared with the experimental data. The predicted axial velocities agree with the experimental data in Figure (4- 11 (d-f)) to within approximately 2% as we move from x= –5 mm to the wall region at negative values of the x-axis. However, moving from x= –5 mm to the wall region at positive values of the x-axis, the simulated profiles are found to under-predict. Summary
The primary focus of this proposal was to design an orifice that focuses the nanoparticles which cross through it. A model was advanced to study the focusing characteristics of sharp plate orifice and was validated against experimental findings. The dependence of focusing of nanoparticles on the aerodynamic lens has been investigated.
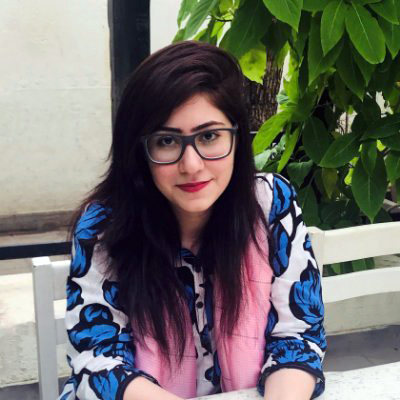
Freelance Writer
I’m a freelance writer with a bachelor’s degree in Journalism from Boston University. My work has been featured in publications like the L.A. Times, U.S. News and World Report, Farther Finance, Teen Vogue, Grammarly, The Startup, Mashable, Insider, Forbes, Writer (formerly Qordoba), MarketWatch, CNBC, and USA Today, among others.